The Gamma probability distribution is very useful to express the presumed prevalence of errors in a population. It has two parameters, a and b, representing shape and scale, respectively. The most likely error is (a-1)b but 0 when a <1; an upper error limit can be derived from the standard Excel function GAMMA.INV(confidence level; a;b). Mean and variance are ab and ab2 respectively.
Graphically, a Gamma distribution can be shaped in many forms: A sample of n items from a population of M monetary units with k errors has a most likely error of k M/n and a maximum error of GAMMA.INV(confidence, k+1, M/n). This makes b to be estimated by M/n and a by k+1.
These formulas can be used to design and evaluate sample results. One detail: the Excel function GAMMA.INV(confidence; a; b) is not always reliable for large values of b and for calculations one should use its scaling property giving GAMMA.INV(confidence; a; 1) x b.
Sample expansion to improve the accuracy of estimated adjustments
Expanding a sample after finding too many errors to a new sample size for which that very number of errors found is acceptable, increases the risk of incorrect acceptance. Therefore, if the original sample size was designed based on tolerated risk, sample expansion will imply that tolerated risk is exceeded and required assurane cannot be achieved.
This implies that when the number of errors exceeds the planned number, sample expansion does not solve the consequence that estimated maximum error exceeds performance materiality. In those situations the amount to be inspected needs to be adjusted before it can be accepted. The most likely error amount is the best candidate for the adjustment amount, but even after that correction, the estimated maximum error may still exceed performance materiality. In that situation, sample expansion can be a good tool to reduce precision, being the distance between the estimated maximum error and the most likely error. This spreadsheet may be helpful.
The sheet yields (by trail and error or by using goal seek) an additional sample size that, assuming it will pick up additional errors in the same fraction as in the first sample, will yield a precise enough estimate of the amount to be adjusted before accepting the population. As a rule of thumb, each additional 100% error can be overcome by 1/3 of sample size.
Consequences of changes in the sampling plan during the year
MUS samples can only be evaluated properly when the interval J = M/n is kept constant over the entire population. Underestimation of the population size therefore may lead to too much work, but adjusting J during the year leads to aggregation problems. Stewart et al.1) have proposed an approximation to the gamma aggregation.
If n1 were selected from M1 with k1 errors and n2 from M2 with k2, we have k1 Gamma distributed with a1 = k1+1 and b1 = M1/n1 yielding an expected value of a1b1 and a variance of a1b1^2 and k2 Gamma distributed with a2 = k2+1 and b2= M2/n2 yielding an expected value of a2b2 and variance a2b2^2 so overall k is approximately Gamma distributed with expectation a1b1+a2b2 and variance a1b1^2 + a2b2^2. This gives us overall b as variance divided by expectation and overall a as expectation divided by b.
As a result we now know the probability distribution of the overall error in two heterogeneous subpopulations. This allows us to aggregate sample results that were sampled with different intervals.
An easier description of the audit risk model
Bayesian inference is based on prior knowledge about the population error that is combined with sample results to draw an overall conclusion.
Combining a prior that is Gamma with parameters a and b with a sample of n from M with k errors, the result is a Gamma posterior with parameter a+k and 1/(1/b+n/M) (re Leonard and Hsu). Given the presumed prior parameters we can use goal seek to determine the sample size (with a number of errors consistent with prior parameters) to achieve a required posterior.
Performance Materiality setting
When a population to be audited consists of two (or more) heterogeneous parts, overall materiality needs to be set for the subpopulations in such a way that if both populations are evaluated with an estimated maximum error below their performance materialities, the aggregated overall estimated maximum error meets the stipulated overall materiality. Materiality setting does not require allocation (sub population performance materialities adding up to overall materiality) since the chance of both errors exceeding their performance materialities is very small: 1-(1-0,05)^n for n 95% upper error limits.
The great advantage of materiality setting is that the assumed heterogeneity allows the evaluation of errors in a subpopulation to be restricted to that subpopulation only. Errors may now be isolated to the sub population were they were found; similar error causes may have been present in other sub populations but if their prevalence is material it will be indicated by sampling on those sub populations.
There is an infinite number of combinations of useful subpopulation performance materialities; T.R. Stewart’s thesis (see page om Bayesian statistics) has shown that square roots or relative sizes are the weights to be applied to sub populations to minimize total sample size.
Audit on an overall population to give assurance on sub populations
The completely opposite situation to materiality allocation is where a homogeneous population is audited, but audit conclusions are required about sub populations and materialities for these sub populations have been set that are lower than overall materiality. Instead of designing samples for each sub population, a two step approach is much more efficient. In the first step an overall sample is designed based on overall materiality. The result of this sample is expressed in a posterior probability function that can be fractionalized into priors for the subpopulations. Each subpopulation receives a Gamma prior, with first parameter a times the relative size of the subpopulation, and b as second parameters. From here, Bayesian models can be built for each sub population to determine the required additional sample.
Note 1) Stewart, T.,R., Strijbosch, L., W. G., Moors, J. J. A., & van Batenburg, P.C. (2007). A Simple Approximation to the Convolution of Gamma Distributions (Revision of DP 2006-27). (CentER Discussion Paper; Vol. 2007-70).
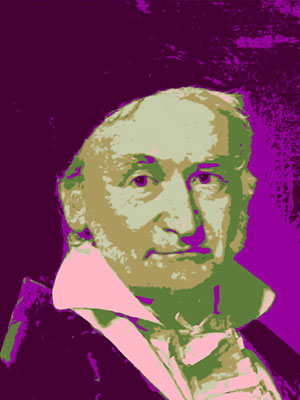
(1777-1855)